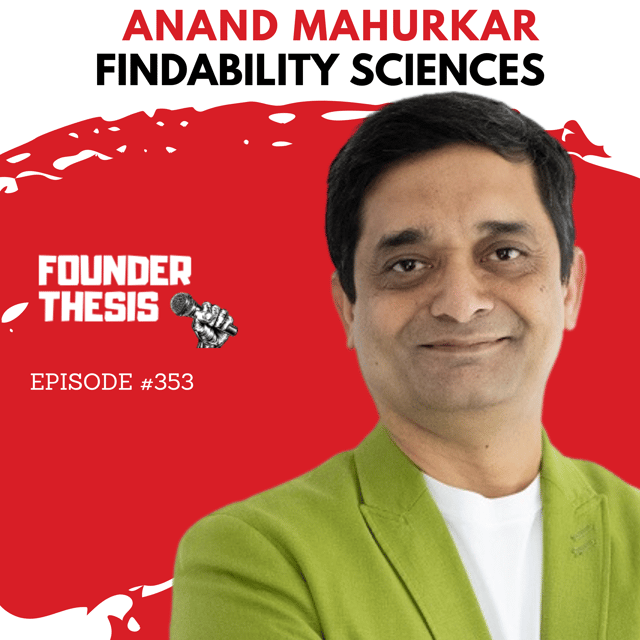
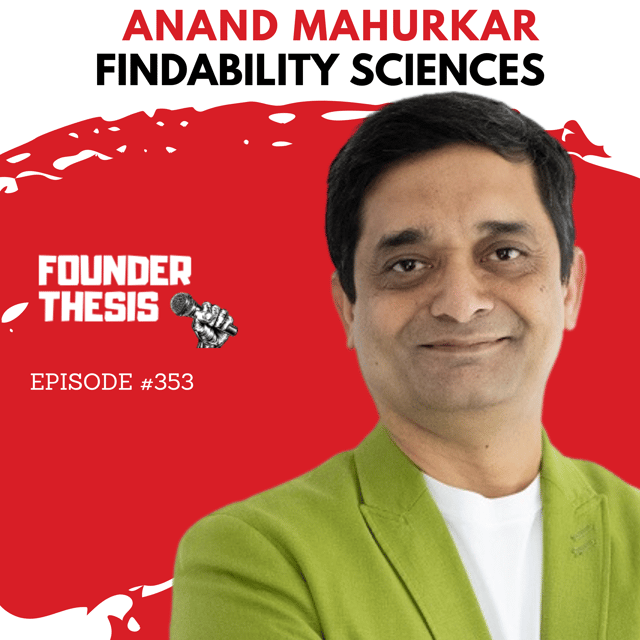
Anand is an IT veteran who started his career three decades back and in his last role was heading an IT services company. Noticing the pain his customers were facing in searching and interpreting data, he recognized the need for a fundamentally new approach to insight generation, which led to the creation of Findability Sciences. In this episode, he talks about how AI is impacting large enterprises and the value it can bring to businesses.
Get notified about the latest releases and bonus content by subscribing to our newsletter at www.founderthesis.com
Read more about Findability Sciences:-
2.Exclusive Interview with Anand Mahurkar, Founder and CEO, Findability Sciences
3.Fortune Magazine recognizes Findability Sciences as one of America's Most Innovative Companies
4.India’s AI roadmap in 2023: From CAGR 20% growth to creating endless job opportunities
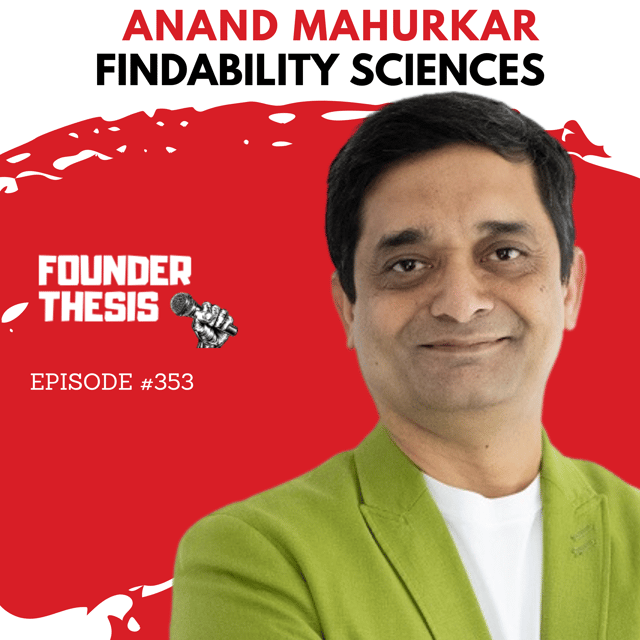
Anand is an IT veteran who started his career three decades back and in his last role was heading an IT services company. Noticing the pain his customers were facing in searching and interpreting data, he recognized the need for a fundamentally new approach to insight generation, which led to the creation of Findability Sciences. In this episode, he talks about how AI is impacting large enterprises and the value it can bring to businesses.
Get notified about the latest releases and bonus content by subscribing to our newsletter at www.founderthesis.com
Read more about Findability Sciences:-
2.Exclusive Interview with Anand Mahurkar, Founder and CEO, Findability Sciences
3.Fortune Magazine recognizes Findability Sciences as one of America's Most Innovative Companies
4.India’s AI roadmap in 2023: From CAGR 20% growth to creating endless job opportunities