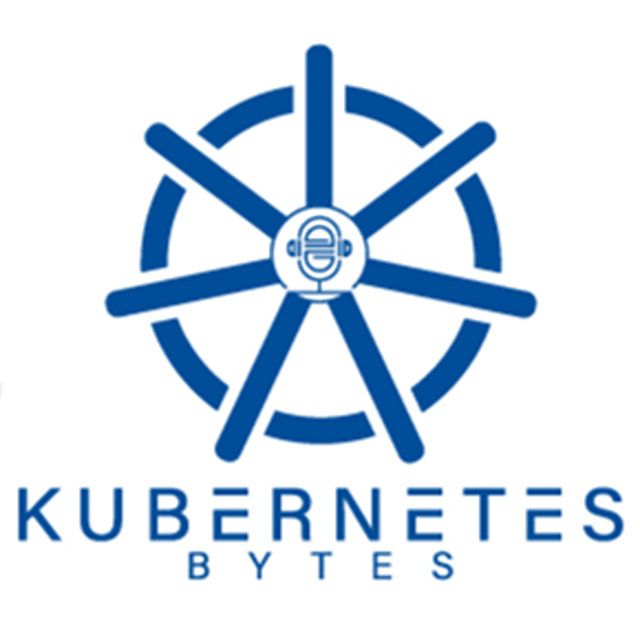
S4 E12 · Kubernetes Bytes
Shifting Minds: Exploring OpenShift's AI Landscape
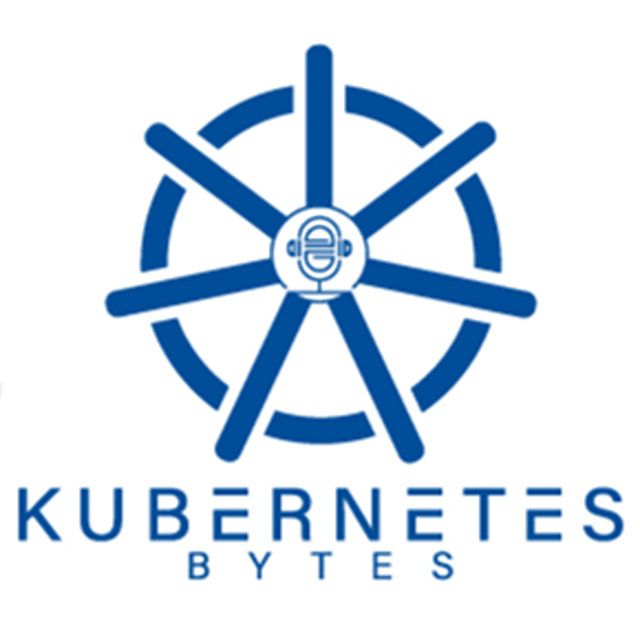
Ryan Wallner and Bhavin Shah talk to Andy Grimes about the OpenShift AI Landscape.
Check out our website at https://kubernetesbytes.com/
Episode Sponsor: Nethopper
- - Learn more about KAOPS: @nethopper.io
- - For a supported-demo: info@nethopper.io
- - Try the free version of KAOPS now! https://mynethopper.com/auth
Links
- - https://youtube.com/watch?v=nAT9U1vJ8x0
- - https://www.theregister.com/2024/06/12/kubertenes_decade_anniversary/
- - https://www.businesswire.com/news/home/20240606882860/en/Mirantis-Collaboration-with-Pure-Storage-Simplifies-Data-Management-with-Kubernetes
- - https://falco.org/blog/falco-0-38-0/
- - https://au.finance.yahoo.com/news/rancher-government-successfully-using-harvester-121100125.html
- - https://www.youtube.com/@PlatformEngineering
- - Video: https://www.youtube.com/watch?v=tZj8j3fdXy4
- - Virtual Road Shows: https://www.redhat.com/en/north-america-red-hat-aws
- - AWS Gameday August 22nd: TBS
- - Boston Childrens Hospital RHOAI: https://www.redhat.com/en/creating-chris
- - IBM Open Source AI https://www.youtube.com/watch?v=SuGedexBudQ&t=141s
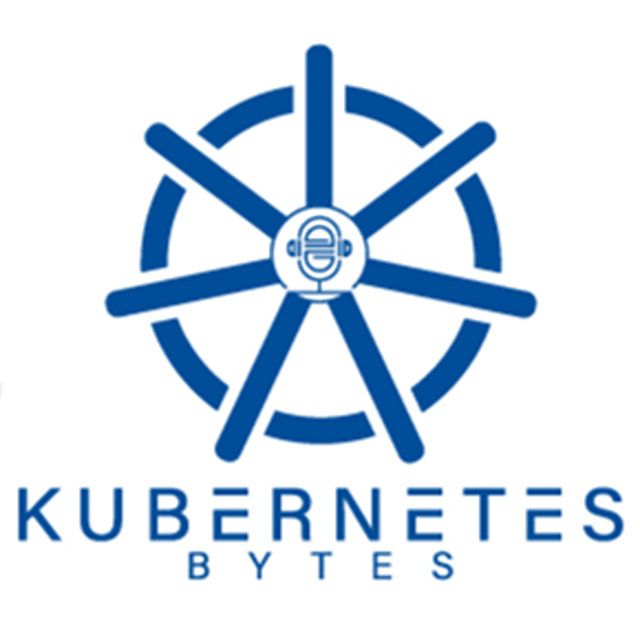
00:00:00
00:00:01
1.2k Plays
3 months agoRyan Wallner and Bhavin Shah talk to Andy Grimes about the OpenShift AI Landscape.
Check out our website at https://kubernetesbytes.com/
Episode Sponsor: Nethopper
- - Learn more about KAOPS: @nethopper.io
- - For a supported-demo: info@nethopper.io
- - Try the free version of KAOPS now! https://mynethopper.com/auth
Links
- - https://youtube.com/watch?v=nAT9U1vJ8x0
- - https://www.theregister.com/2024/06/12/kubertenes_decade_anniversary/
- - https://www.businesswire.com/news/home/20240606882860/en/Mirantis-Collaboration-with-Pure-Storage-Simplifies-Data-Management-with-Kubernetes
- - https://falco.org/blog/falco-0-38-0/
- - https://au.finance.yahoo.com/news/rancher-government-successfully-using-harvester-121100125.html
- - https://www.youtube.com/@PlatformEngineering
- - Video: https://www.youtube.com/watch?v=tZj8j3fdXy4
- - Virtual Road Shows: https://www.redhat.com/en/north-america-red-hat-aws
- - AWS Gameday August 22nd: TBS
- - Boston Childrens Hospital RHOAI: https://www.redhat.com/en/creating-chris
- - IBM Open Source AI https://www.youtube.com/watch?v=SuGedexBudQ&t=141s
Recommended