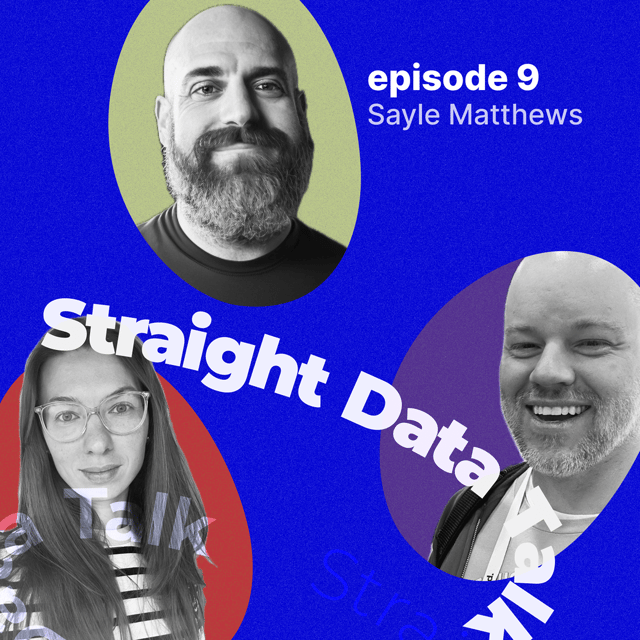
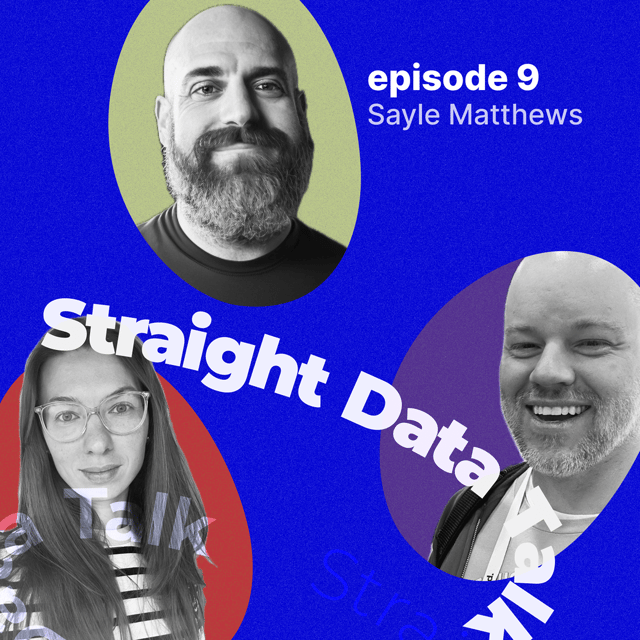
Sayle Matthews leads the North American GCP Data Practice at DoiT International. Over the past year and a half, he has focused almost exclusively on BigQuery, helping hundreds of GCP customers optimize their usage and solve some of their biggest 'Big Data' challenges. With extensive experience in Google BigQuery billing, we sat down to discuss the changes and, most importantly, the impact these changes have had on the market, as observed by Sayle while working with hundreds of clients of various sizes at DoiT.
Sayle's LinkedIn page - https://www.linkedin.com/in/sayle-matthews-522a795/
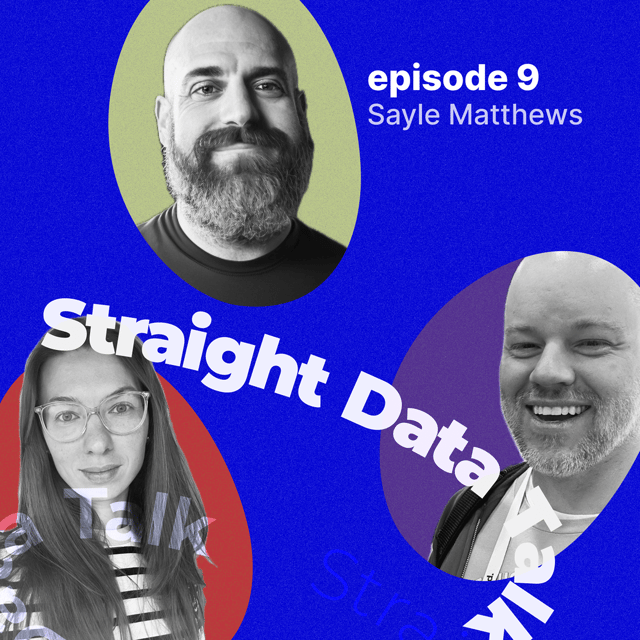
Sayle Matthews leads the North American GCP Data Practice at DoiT International. Over the past year and a half, he has focused almost exclusively on BigQuery, helping hundreds of GCP customers optimize their usage and solve some of their biggest 'Big Data' challenges. With extensive experience in Google BigQuery billing, we sat down to discuss the changes and, most importantly, the impact these changes have had on the market, as observed by Sayle while working with hundreds of clients of various sizes at DoiT.
Sayle's LinkedIn page - https://www.linkedin.com/in/sayle-matthews-522a795/