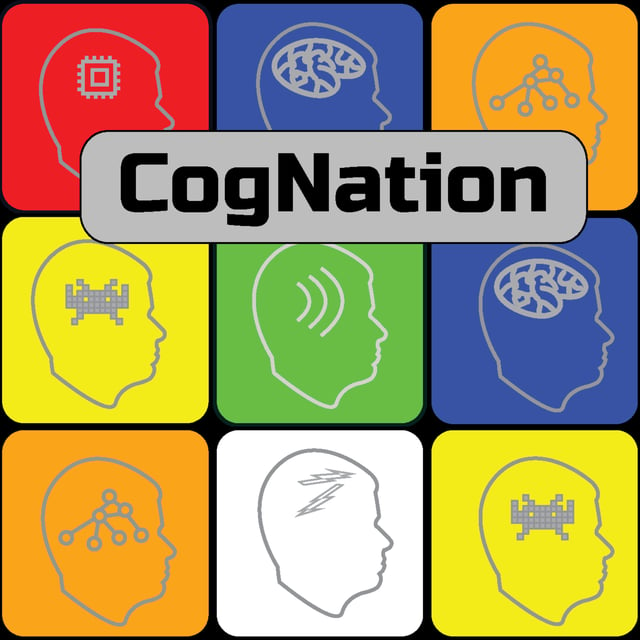
S1 E16 · CogNation
Episode 16: Dopamine with Michael Frank
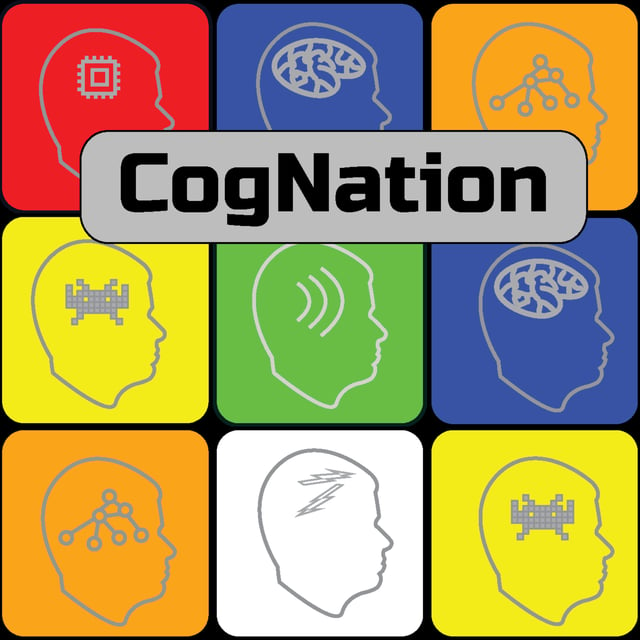
Dr. Michael Frank of Brown University talks to us about dopamine -- how it works in the brain, what his research has done to elucidate the function of dopamine circuits, and some of the genetics behind it. A really fascinating dive into a great topic!
Papers:
Dopamine and free will:
Dopamine and learning:
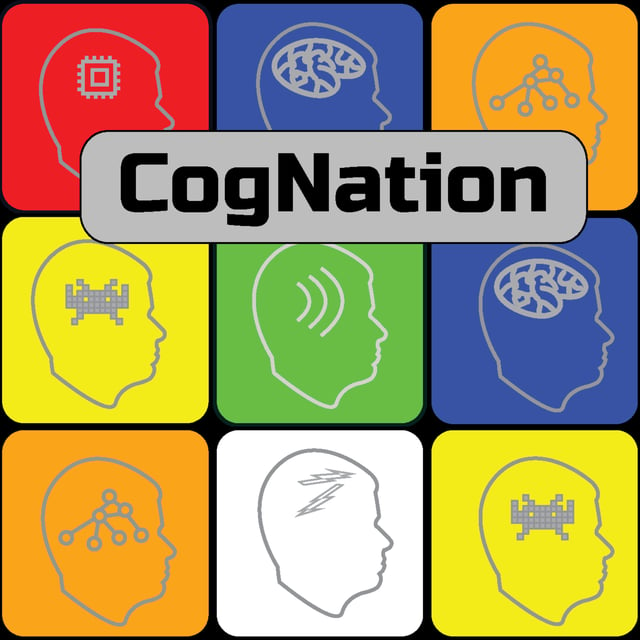
00:00:00
00:00:01
14 Plays
5 years agoDr. Michael Frank of Brown University talks to us about dopamine -- how it works in the brain, what his research has done to elucidate the function of dopamine circuits, and some of the genetics behind it. A really fascinating dive into a great topic!
Papers:
Dopamine and free will:
Dopamine and learning:
Recommended