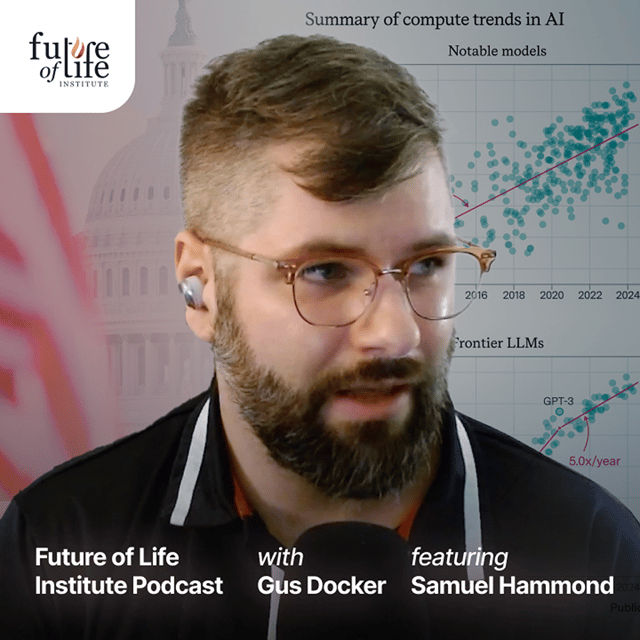
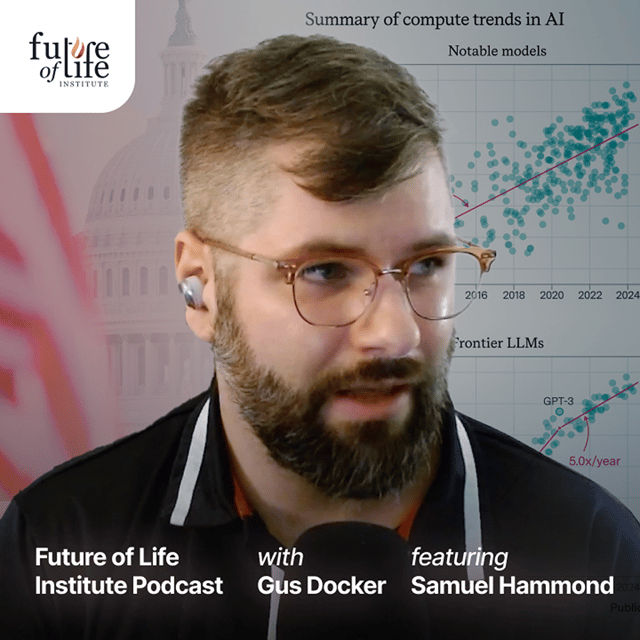
Samuel Hammond joins the podcast to discuss whether AI progress is slowing down or speeding up, AI agents and reasoning, why superintelligence is an ideological goal, open source AI, how technical change leads to regime change, the economics of advanced AI, and much more.
Our conversation often references this essay by Samuel: https://www.secondbest.ca/p/ninety-five-theses-on-ai
Timestamps:
00:00 Is AI plateauing or accelerating?
06:55 How do we get AI agents?
16:12 Do agency and reasoning emerge?
23:57 Compute thresholds in regulation
28:59 Superintelligence as an ideological goal
37:09 General progress vs superintelligence
44:22 Meta and open source AI
49:09 Technological change and regime change
01:03:06 How will governments react to AI?
01:07:50 Will the US nationalize AGI corporations?
01:17:05 Economics of an intelligence explosion
01:31:38 AI cognition vs human cognition
01:48:03 AI and future religions
01:56:40 Is consciousness functional?
02:05:30 AI and children
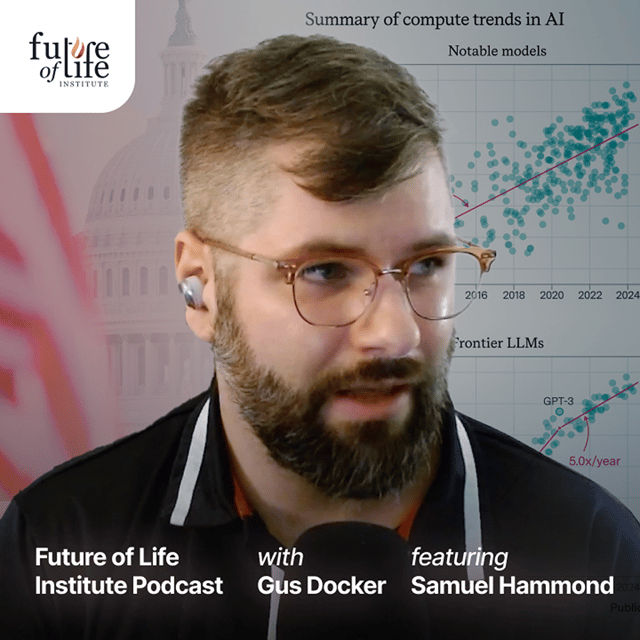
Samuel Hammond joins the podcast to discuss whether AI progress is slowing down or speeding up, AI agents and reasoning, why superintelligence is an ideological goal, open source AI, how technical change leads to regime change, the economics of advanced AI, and much more.
Our conversation often references this essay by Samuel: https://www.secondbest.ca/p/ninety-five-theses-on-ai
Timestamps:
00:00 Is AI plateauing or accelerating?
06:55 How do we get AI agents?
16:12 Do agency and reasoning emerge?
23:57 Compute thresholds in regulation
28:59 Superintelligence as an ideological goal
37:09 General progress vs superintelligence
44:22 Meta and open source AI
49:09 Technological change and regime change
01:03:06 How will governments react to AI?
01:07:50 Will the US nationalize AGI corporations?
01:17:05 Economics of an intelligence explosion
01:31:38 AI cognition vs human cognition
01:48:03 AI and future religions
01:56:40 Is consciousness functional?
02:05:30 AI and children